Course Information
This is a project-based class where students will learn how to develop machine learning models for execution in resource constrained environments such as embedded systems. The primary target is embedded devices such as Arduino, Raspberry PI, Jetson, or Edge TPUs.
The class is broken into lectures/readings, labs/assignments, and a final project. Throughout this class, students will learn techniques such as model quantization, knowledge distillation and hybrid (embedded/cloud inferencing), which are instrumental for building efficient machine learning models that can run on power or resource-constrained devices. This class differs from other machine learning classes offered at Stanford due to the focus of applying these models for applications that require running on embedded hardware or other resource-constrained environments.Teaching Staff
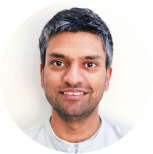
Zain Asgar
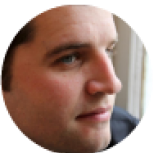
Pete Warden
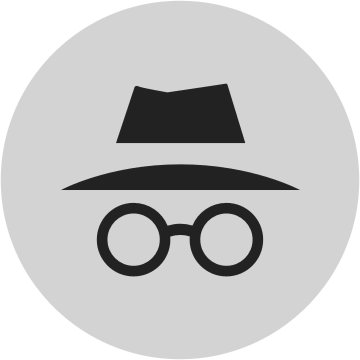
Sachin Katti
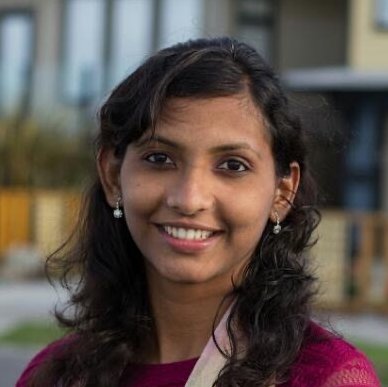
Ankita Nayak
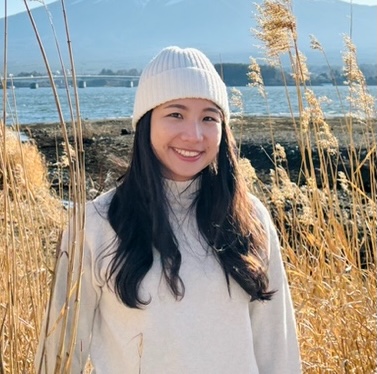
Yi-Chin Huang
Course Schedule
Week 1 |
Intros, objectives, etc.
Labs
|
Week 2 |
Introduction to CNN and tranformer models
Labs
|
Week 3 |
ML model training and fine-tuning techniques
Labs
|
Week 4 |
Finding and evaluating datasets
Labs
|
Week 5 |
ML model optimization
Labs
|
Week 6 |
ML model training in practice
Labs
|
Week 7 |
Guest lecture
Labs
|
Week 8 |
Hardware for DNN
Labs
|
Week 9 |
MLOps
Project
|
Lab Assignments
Lab Assignment 1: Set up your Raspberry Pi
This tutorial shows how to set up your laptop and Raspberry Pi 5 board to run machine learning labs in EE292D.
Lab Assignment 2: Run a Large Language Model
This lab will show you how to run an LLM locally on your Raspberry Pi 5.
Lab Assignment 3: Classify Images
This lab will show you how to run an image classification model on your Pi, and fine-tune it to recognize different kinds of objects.
Lab Assignment 4: Locate People and Objects
This lab will show you how to run pretrained models to spot people, analyze their poses, and locate arbitrary objects in images.
Lab Assignment 5: Recognize and Generate Speech
This lab will show how to run an open source speech to text model on your Pi, connect it to your large language model, and speak the answers.
Lab Assignment 6: Fine Tune Large Language Model
This lab will show you how to fine tune an LLM for a custom task and run it on your Pi.
Grading
Labs - 30%
- ~5 labs that we will work through in the second class each week
Reading Assignments/Class Participation - 10%
- Reading Reflections
- Lead group discussions on each paper
- Graded on participation and write-ups
Final Project - 60%
- Proposal - 10% (2% for initial, 8% for final)
- Write up & Presentation - 30%
- Results - 20% (relative to proposed)
Important Dates
Date | What's Due | |
---|---|---|
Week 2 (April 12) | Preliminary final project proposal due | |
Week 3 (April 19) | Final project proposal due | |
Week 7 (May 15) | In class show and tell about project progress | |
Week 9 (May 29) | Final project presentations due (last class) | |
Week 9 (May 31) | Final project report due |